What drives the M3 project and what exactly are we trying to learn?
Background
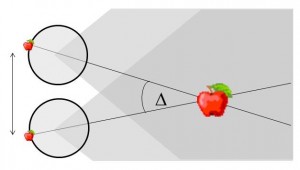
Figure 1. The basic principle of stereo 3D vision. If an object is viewed by two eyes, its location can be deduced by triangulating back from the two images. Its distance depends on the binocular disparity Delta.
Our rich visual experience is constructed within our heads by the electrical activity of brain cells or neurons. How exactly neural tissue can convert a stream of photons on the retina into visual perception has long occupied scientists and philosophers. In recent decades, stereo 3D depth perception has emerged as a particularly useful model system with which to probe the neuronal computations underlying perception. 3D vision refers to our ability to detect binocular disparities between the position of an object’s image in the two eyes, and triangulate back from this to deduce the object’s distance from us. This stereo depth perception overcomes a fundamental limitation of vision: that an eye collapses a 3D world onto a 2D light-sensing surface. Remarkably, despite research on binocular vision dating back to the tenth-century scholar Ibn al-Haytham, 3D vision was discovered only 170 years ago. Thanks to breakthroughs in digital technology, our stereo depth perception is now being exploited in new 3D movies, TV, games and even mobile phones. 3D displays have applications from computer-aided design to medical imaging. A crucial advantage of 3D vision is that the underlying geometry is straightforward and independent of the particular scene viewed (Fig 1). Engineers have produced computer algorithms which give robots 3D vision, while neuroscientists have uncovered similar computations in various areas of the primate brain. However, machine 3D vision struggles with tasks which humans solve effortlessly, such as complex natural scenes with “lacy” structure like tree branches. And although modern techniques have revealed many of the brain areas which contribute to primate 3D vision, the neuronal circuits involved are simply too complex for us to reconstruct using currently-available techniques.
3D vision in animals
This research programme tackles 3D vision in a far simpler organism: the praying mantis. Insect brains consist of only around a million neurons, compared to the tens of billions in the human brain; the difference in the number of connections between neurons is greater still. In insects, some large neurons can even be identified reliably from individual to individual: one can identify a particular fly’s H5 neuron in the same way as one can identify its right antenna. The relative simplicity of the insect system means that the circuitry can be traced much more easily. For example, one can realistically seek to identify all inputs to a given neuron. Connections between different brain areas can be traced by filling and reconstructing individual neurons. Modern genetic and optogenetic techniques are enabling increasingly sophisticated interventions, e.g. reversibly inactivating all neurons of a particular class.
3D vision has been demonstrated in many animals, including monkey, cat, owl, falcon, toad, and horse. But until 1983, many biologists believed that invertebrate brains were too simple to see in 3D. That changed with the demonstration of 3D vision in the praying mantis (refs 39). The mantis seems physically optimised for 3D vision. It has a triangular head to maximise the 3D vision baseline, a massive binocular overlap of >70deg, and a forward-facing fovea – a specialised region of high visual acuity – in each of its compound eyes. Its lifestyle would surely be aided by 3D vision: it is an ambush predator which lives in a cluttered visual environment of dense vegetation and eats highly camouflaged prey such as crickets. It catches this prey with a dramatic strike of its spiked forelegs which lasts just 40ms, so an accurate judgment of the prey’s distance is critical. To test how mantids judge distance, Samuel Rossel (ref 39) placed prisms over their eyes. This alters the binocular disparity without affecting other cues such as object size. The mantids struck at the object when its apparent disparity indicated it was within range, independent of its actual distance. Together, Rossel’s series of experiments (refs 39-43) proved convincingly that mantids have 3D vision.
We now know that 3D vision has evolved independently at least four times: in mammals, birds, amphibians and insects. 3D vision has been investigated thoroughly in two non-human species, barn owls and macaque monkeys. In contrast, mantis 3D vision has not been investigated in any detail. Even its function is not clear. We know that it aids the predatory strikes by enabling precise judgments of near distances, but there is also suggestive evidence that mantids may use their 3D vision to judge much larger distances. This would help them, for example, discriminate a small bird nearby from a larger, predatory bird further away.
Different kinds of 3D vision: “range-finding” versus “camouflage-breaking”
In humans, 3D vision has much wider benefits. As early as the First World War, it was discovered that camouflaged military structures became easy to pick out if aerial reconnaissance photographs were viewed in 3D in a stereoscope. Objects which match their backgrounds in colour and brightness are hard to detect with one eye, but jump out in depth if viewed in 3D. Thus, our 3D vision is said to “break camouflage”. Artificial images can be created where objects are completely invisible with one eye, but pop out in 3D. Even without camouflage, “scene segmentation” – figuring out where one object stops and another begins – is a hard problem in computer vision, which becomes much easier using 3D.
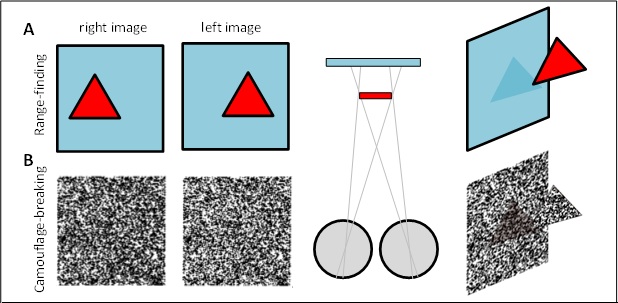
Figure 2. (A) Range-finding vs (B) camouflage-breaking 3D. In both A and B, the images depict a triangle in front of a background. In A, the triangle is visible in each eye individually. B shows a random-dot stereogram37. Here, each eye sees featureless noise, with no triangular object visible. But viewed binocularly, a triangle pops out in depth. Readers who can manage to cross their eyes so that each eye views the appropriate image will be able to see this effect for themselves.
All current machine 3D algorithms break camouflage automatically, as a side-effect of how they achieve robust performance. Every 3D-capable animal species which has been tested has also been able to break camouflage. The 3D vision of macaque monkeys and barn owls has been probed in detail, and appears functionally identical to our own in every respect, including the ability to break camouflage. This is remarkable given that primate and bird stereo evolved independently to suit very different lifestyles, eyes and neuroanatomy (44). Apparently avian, mammalian and computer 3D vision have all converged on the same basic solution. This has led to the conclusion that the properties of natural images, and the geometry of binocular vision, must place fundamental constraints on any 3D visual system. These ecological and geometrical constraints mean that any useful 3D system must employ essentially the same basic algorithm, including the ability to break camouflage (44). Indeed, camouflage-breaking is now viewed as a key reason why 3D vision evolved in vertebrates (44, 45).
In contrast, most invertebrate biologists do not believe that insect 3D vision can possibly be capable of breaking camouflage (46, 47). Given their simple nervous systems, and the small number of binocular neurons so far identified in insect brains, it is assumed that mantis 3D vision must work in a completely different way. For example, mantids may identify a single target in each eye individually, and then compute the disparity of that target, without comparing the two eyes’ images to see if the targets match (40). This comparison, known as correspondence, is a key step in all known 3D algorithms, so it would be remarkable if mantid vision does indeed skip it and still achieves robust performance in complex natural scenes.
From individual neurons to animal behaviour
Amazingly, since Rossel’s pioneering work, no further research has been done on insect 3D vision. The intervening decades have seen major advances in our understanding of how neuronal activity relates to perception and to behavioural decisions, in which primate 3D vision has played a key role. Over the same period, invertebrate neuroscience has spearheaded the development of techniques which make it possible to understand neuronal circuits in unprecedented detail. Insect 3D vision offers a way for these advances from different areas of neuroscience to be combined, ultimately resulting in a complete algorithmic account of behavioural decisions at the level of individual neurons. Mantis 3D vision would be the most complex perceptual ability yet understood at this level of detail.
Main aims of the research
To date, virtually nothing is known about insect 3D. This research programme will combine behavioural, computational and electrophysiological techniques to revolutionise our understanding of insect 3D. We will:
- Fully characterise mantid 3D vision.
- Establish whether mantis 3D vision is functionally equivalent to that of mammals and birds.
- Discover cells in the mantid nervous system which encode binocular depth.
- Characterise the response of neurons at different points along the pathway from phototransduction to movement.
- Develop computer models which describe the responses of individual neurons.
- Develop computer models relating neuronal activity to behaviour in the mantid.
This will:
- Reveal whether 3D vision admits only one basic solution.
- Shed new light on why and how 3D vision evolved.
- Produce a robust and useful machine 3D algorithm of unprecedented efficiency.
- Help understand perception and behaviour in humans and other vertebrates.
Research Plan
Methods
Study subjects
Mantids are ideal organisms to study. I have argued above that their simpler nervous system offers substantial advantages over non-human primates. Critically, they also retain some of the key experimental advantages of primates. Because monkeys can be trained to report their visual perceptions, electrophysiological recording can be combined with simultaneous perceptual judgments, enabling fluctuations in neuronal activity to be correlated with variation in perception. Without training, mantids’ natural behaviour effectively reports many aspects of their visual perception. Mantids turn their heads to track moving objects, and strike at nearby objects that resemble prey (39-43, 48, 49). So in our behavioural experiments, measuring their head movements will tell us what they can see. For electrophysiology, we will need to immobilise the head. Conveniently, head-fixed mantids still continue to strike, even if not rewarded by prey capture (40, 41, 49). By measuring the strike, we can find out where in space they perceived the object. This means that we can combine electrophysiological recordings with simultaneous measurements of depth judgments. This powerful approach has enabled major progress in primate vision, but has not previously been applied to insect 3D. Mantids also offer some more technical advantages, e.g. the fact that they cannot move or focus their compound eyes simplifies the interpretation of 3D vision experiments and avoids the complex eye-tracking required in primates (15).
The pioneering behavioural experiments on mantis 3D vision were conducted largely by hand39-43. Even when behaviour was recorded with video camera, the footage still had to be painstakingly analysed by hand. The time-consuming nature of the experiments limited the data available. A key feature of this research programme will be the exploitation of modern digital technology. We will develop automated 3D display, data collection and analysis, enabling rapid collection of thousands of trials. The statistical power unlocked by these novel approaches will make it possible to quantify small changes in strike probability which would otherwise be undetectable.
Visual stimulation
Visual images will be generated by the computer on each trial, enabling fast, flexible experiments, in which many different types of stimuli can be randomly interleaved. This will require us to develop the first 3D display for insects. We plan to use circular polarisation to separate the images viewed by the two eyes, as in many types of 3D TVs and computer monitors. (Insect eyes are sensitive to linear polarisation, but not circular.) We will create “mantid 3D glasses” by placing circularly-polarising filters in front of each eye instead of the prisms used by Rossel (39-43).
Behavioural recordings
Two high-speed video cameras will record the mantid’s position from below and from the side. These will interface with the main experiment computer so that after each trial, the computer will download the image sequences from each camera showing the mantid’s behaviour during that trial, and will save them to disk along with the parameters of the visual stimulus. High-speed cameras recording at 1000 frames per second will ensure that we capture the fine detail of the mantis strike.
In offline analysis, a computer program will automatically analyse these image sequences and convert them into quantitative measurements of behaviour. For example, the mantid’s response will be classified as strike/tracking/evasion/freezing, etc. For experiments where the animal’s head is free, it will be important to measure head position and thus know what the animal is looking at. For strike responses, we will usually want to extract the time at which a strike was released relative to stimulus onset, and hence the apparent distance of the target at strike release, and the 3D vision location at which the strike was directed. Reflective paint spots dabbed onto the mantid’s head and joints will provide easily identifiable targets for our analysis algorithms.
We will be aided by expertise from Dr Candy Rowe and Dr Geraldine Wright, colleagues here at Newcastle who carry out behavioural experiments on insects including praying mantids.
Electrophysiology
We will record extracellular activity in the mantis nervous system, measuring the electrical “spikes” which are produced by a subset of neurons. We will work with Dr Claire Rind and Dr Peter Simmons, colleagues who are world-leaders in insect electrophysiology, and also with their long-term collaborator Dr Yoshifumi Yamawaki at Kyushu University, who carries out behavioural and electrophysiological recording in mantids. We will employ the silver wire electrodes successfully used by Dr Rind in locust, and by Dr Yamawaki in mantis (50-53), and also multi-channel electrodes (e.g. Tetrodes) to maximise data acquisition. In later years of the project, we will record from two or more locations at once, e.g. in optic lobe and in ventral cord, in order to uncover correlations between neuronal activity in different locations.
Experimental projects
The function and capabilities of mantis 3D vision
We will establish whether mantis 3D vision is capable solely of range-finding or also of camouflage-breaking: detecting prey which is invisible to each eye in isolation. Dynamic noise images resembling those in Fig 2B will depict a small object making jerky movements, which we know attract mantids’ attention and cause them to turn their heads to track the object as it moves across the screen (48). In such stimuli, the images change randomly from moment to moment, so each eye individually sees only noise, without any coherent motion. But viewing with both eyes, humans and monkeys see the moving object standing out in depth (12). If mantids never make head movements towards such targets, even after we have explored a large range of different stimulus types (contrast, type of movement, size of texture elements etc), we will conclude that mantis 3D vision cannot break camouflage. This will imply that mantis 3D vision evolved purely for range-finding on already-identified objects, rather than also for detecting these objects in the first place.
Neurons tuned to disparity
Having established what mantis 3D vision is capable of, we will begin uncovering the neurons responsible. Vertebrate 3D vision requires neurons sensitive to binocular disparities between the eyes (Delta in Fig 1). No such neurons are yet known in the mantis, so a major aim of this project will be to identify them. Fig. 3 indicates some of the key brain areas we plan to record from. After phototransduction in the compound eye, visual information is processed in the optic lobe, initially in the lamina and subsequently in the medulla and lobula. The mushroom bodies appear to combine information from different sensory modalities. We will record in all these areas. We will also probe specific mantid neurons already known to be tuned to related aspects of vision. For example, Dr Yamawaki has shown that mantis ventral cord contains neurons which respond to “looming”, i.e. they fire when the animal views an object progressively increasing in size, as if approaching (53). We will test whether these neurons also respond to motion in depth when this is indicated by changes in binocular disparity.
The correspondence problem
At the heart of any 3D algorithm is correspondence: figuring out which point in the left eye’s image corresponds to the same location in space as a given point in the right. A unique feature of stereo vision is that one can artificially create pairs of images where no solution exists, which can never occur in nature. Measuring a system’s response to such impossible images provides vital information about its 3D algorithm, a fact I have exploited in my work on primate 3D vision (3,17). In the monkey, as we follow visual information through successive brain areas, the neuronal response to impossible images progressively decreases, presumably representing successive steps in the algorithm (11). We will measure the mantid’s behavioural and neuronal response to these impossible images, thereby tracking how 3D information is transformed as it moves through successive levels of the nervous system, e.g. the layers of the optic lobe. In the monkey, I (3-6,8,17) and others have produced models of the possible algorithmic steps, but the complexity of the system makes it hard to test these. For example, one of my models predicts inhibitory connections between two particular classes of disparity-tuned neurons (17). Such predictions are hard to test in primate because of the low probability of finding connected cell-pairs amongst millions of neurons. In an insect, the odds of finding such connections are many orders of magnitude better.
Relating neuronal activity to behaviour
Recent years have seen major steps in understanding the neuronal basis of behavioural decisions in the monkey. Information-theoretic “diffusion-to-bound” models, originally developed to break enemy codes in World War II, account remarkably well for the monkey’s perceptual decisions and reaction times. Neurons in monkey parietal cortex behave as predicted by these models, accumulating noisy sensory evidence until a required level of confidence is reached (54). However, the significance of such activity is hotly debated. If neuronal activity in a given cortical area correlates with the animal’s decisions, does this indicate that the neuronal activity is causing the decision? Or is it merely reflecting a decision made elsewhere? Because of the huge size of the monkey brain, it is not possible to trace how these signals are generated.
In the mantid nervous system, this should be easier. The mantid’s strike provides a convenient example of a behavioural decision; as noted above, mantids continue to strike at potential prey objects even when their head and thorax are immobilised. We will correlate neuronal activity with properties of the visual image, such as contrast or binocular disparity (Delta in Fig 1), and with striking behaviour. In early computational stages, e.g. in the optic lobe, we expect neuronal activity to depend on what the animal is seeing rather than how it is behaving. At the other end, motoneuron activity must encode the striking behaviour, regardless of what the animal saw to trigger the strike. In between, we expect to find neurons whose activity depends partly on the visual images and partly on the behavioural response. While insects may not experience perception as primates do, activity in such neurons is still a valuable analogue of computations underlying perception in more complex organisms.
A new stereo algorithm
The knowledge gained from all these experiments will be combined to produce a computer model of mantis 3D vision, closely based on extracellular neurophysiology. The model will begin with the retinal images, transformed by the optics of the compound eye. Our measurements of binocular neurons will be used to model how these images are processed to produce an initial encoding of binocular disparity. We may find that interocular cross-correlation works well, as it does in the primate, or we may have to turn to a completely different approach, such as feature-based encoding (40). Our results concerning correspondence will constrain how the algorithm detects and rejects false matches between the two eyes’ images. The result will be a machine 3D algorithm which takes binocular video streams as input, and transforms them into behavioural outputs such as fixate, track and strike. These could be used to control a robot (55, 56), with the appropriate changes to behaviour (e.g. “strike” might correspond to “pick up”). This algorithm may be simpler than existing machine algorithms, and yet may outperform them.
Further reading
Bold papers are especially relevant. My own papers are available here.
3. Read JCA & Eagle RA (2000) Reversed stereo depth and motion direction with anti-correlated stimuli. Vision Research 40: 3345-3358
4. Read JCA (2002) A Bayesian model of stereo depth / motion direction discrimination. Biological Cybernetics 82: 117-136
5. Read JCA (2002) A Bayesian approach to the stereo correspondence problem. Neural Computation 14:1371-1392
6. Read JCA, Parker AJ, Cumming BG (2002) A simple model accounts for the response of disparity-tuned V1 neurons to anti-correlated images. Visual Neuroscience 19: 735-753
8. Read JCA, Cumming BG (2003) Testing quantitative models of binocular disparity selectivity in primary visual cortex. Journal of Neurophysiology 90: 2795-2817
11. Read JCA (2005) Early computational processing in binocular vision and depth perception. Progress in Biophysics and Molecular Biology, 87: 77-108.
17. Read JCA, Cumming BG (2007) Sensors for impossible stimuli may solve the stereo correspondence problem. Nature Neuroscience, 10: 1322-1328
37. Julesz (1960) Binocular depth perception of computer-generated patterns. Bell System Technical Journal, 39: 1125-62.
39. Rossel (1983) Binocular stereopsis in an insect. Nature, 302: 821-822.
40. Rossel (1986) Binocular Spatial Localization in the Praying-Mantis. Journal of Experimental Biology, 120: 265-281.
41. Rossel (1991) Spatial Vision in the Praying-Mantis – Is Distance Implicated in Size Detection. Journal of Comparative Physiology A, 169: 101-108.
42. Rossel (1996) Binocular vision in insects: How mantids solve the correspondence problem. Proceedings of the National Academy of Sciences of the United States of America, 93: 13229-13232.
43. Rossel, et al. (1992) Vertical Disparity and Binocular Vision in the Praying-Mantis. Visual Neuroscience, 8: 165-170.
44. Pettigrew (1991) Evolution of binocular vision, in The evolution of the eye and visual system, J.R. Cronly-Dillon and R.L. Gregory, Editors, CRC Press. p. 271-83.
45. van der Willigen (2011) Owls see in stereo much like humans do. Journal of Vision, 11(7):10, 1-27.
46. Collett (1996) Vision: Simple stereopsis. Current Biology, 6: 1392-1395.
47. Schwind (1989) Size and distance perception in compound eyes, in Facets of Vision, Stavenga & Hardie, Eds. p. 425-444.
48. Prete, et al. (2011) Visual stimuli that elicit appetitive behaviors in three morphologically distinct species of praying mantis. Journal of Comparative Physiology A, 197: 877-894.
49. Mittelstaedt (1957) Prey capture in mantids, in Recent advances in invertebrate physiology, B. Scheer, et al., Eds., University of Oregon Publications: Oregon p. 51-71.
50. Yamawaki & Toh (2003) Response properties of visual interneurons to motion stimuli in the praying mantis, Tenodera aridifolia. Zoological Science, 20: 819-832.
51. Yamawaki & Toh (2005) Responses of descending neurons in the praying mantis to motion stimuli. Zoological Science, 22: 1477-1478.
52. Yamawaki & Toh (2009) A descending contralateral directionally selective movement detector in the praying mantis Tenodera aridifolia. Journal of Comparative Physiology A, 195: 1131-1139.
53. Yamawaki & Toh (2009) Responses of descending neurons to looming stimuli in the praying mantis Tenodera aridifolia. Journal of Comparative Physiology A, 195: 253-264.
54. Gold & Shadlen (2007) The neural basis of decision making. Annual Review of Neuroscience, 30: 535-74.
55. Arkin, et al. (2000) Behavioral models of the praying mantis as a basis for robotic behavior. Robotics and Autonomous Systems, 32: 39-60.
56. Bruckstein, et al. (2005) Head movements for depth perception: Praying mantis versus pigeon. Autonomous Robots, 18: 21-42.